We believe we can
inspire all data owners to open their data for research
by building open-source privacy software that empowers them to receive more benefits (co-authorships, citations, grants, etc.) while mitigating risks related to privacy, security, and IP.
Researchers need data they cannot access
Billions of mammography images exist globally, but the largest breast cancer dataset has under 4 million images. Without more data, AI detection is less effective than radiologist’s manual review. Connecting non-public data with researchers could improve detection. Many similar problems exist.
Data owners have data they cannot share
Traditionally, data owners must share their data with researchers to aid in research, but they often can’t due to privacy, security, or IP concerns. As a result, groundbreaking research is blocked, and data owners miss out on potential rewards like co-authorships, citations, and grants.
A second wave of big data is coming
The Syft network makes it possible for a researcher in one organization to conduct research on data in another — without acquiring a copy. This means researchers can use Syft to query far more data than ever before.
Join the movement
Syft is trusted by leading global organizations, enabling unparalleled opportunities for collaborative research, authorship, citations, and grants.
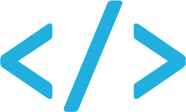
Need more data?
Quickstart Guide
Learn how to get or grant privacy-enhanced access to non-public data using Syft.
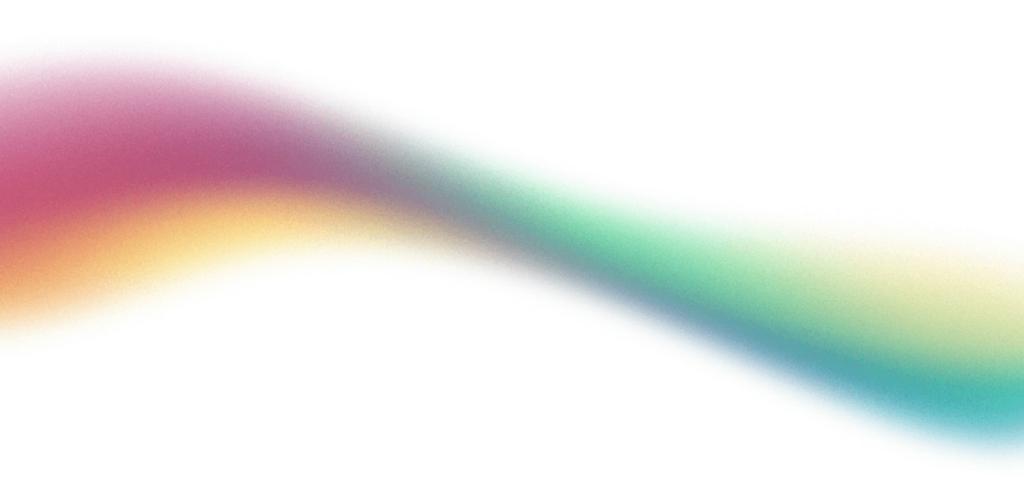
Introducing Syft: The Public Network for Non-Public Information
Syft allows external researchers to securely conduct remote data science while protecting privacy, security, and intellectual property. With advanced privacy-enhancing technologies (PETs), Syft allows researchers to get answers hidden in private or sensitive data without making a copy or allowing direct access.
Free & Open Source Software for Data Scientists
Built and maintained by the OpenMined Foundation and Community, Syft runs on free, open source software called PySyft.
Responsible Data Access Infrastructure
Syft uses secure ‘datasite’ servers to keep sensitive data inaccessible while enabling remote data science on mirrored ‘mock data’.
A network, like the internet, but for non-public data
The Syft Network connects ‘datasite’ servers globally, enabling organizations to collaborate on research and projects across securely stored data while mitigating privacy & IP risks.
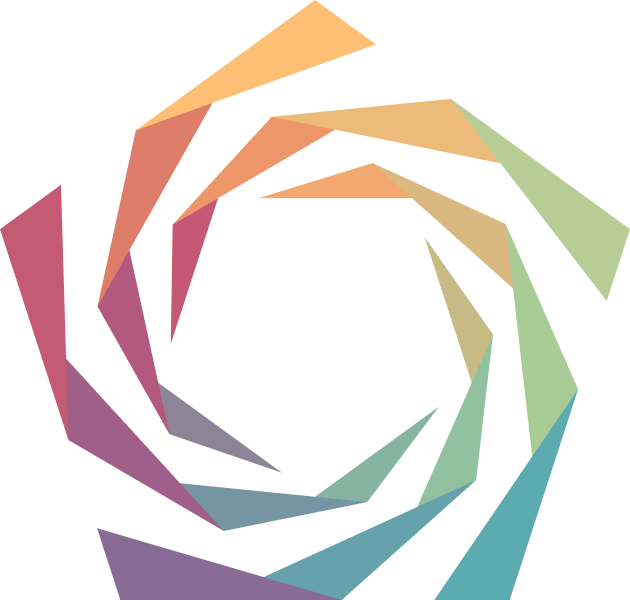
1,000x more research in every scientific field and industry is possible.
How it works
Find a Datasite
Browse available datasites or collaborate with a data owner to launch a new one.
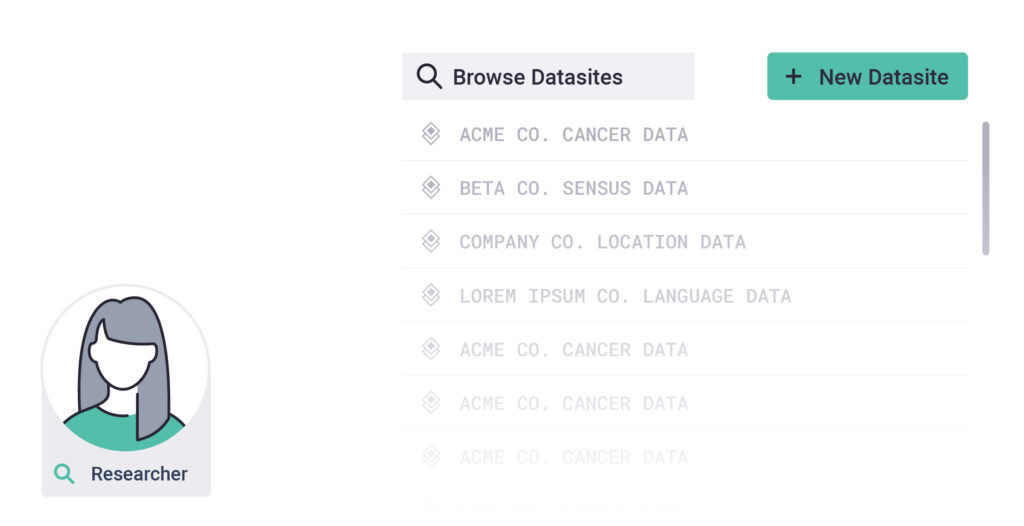
Submit a Research Proposal
Send your code to the datasite owner for review.
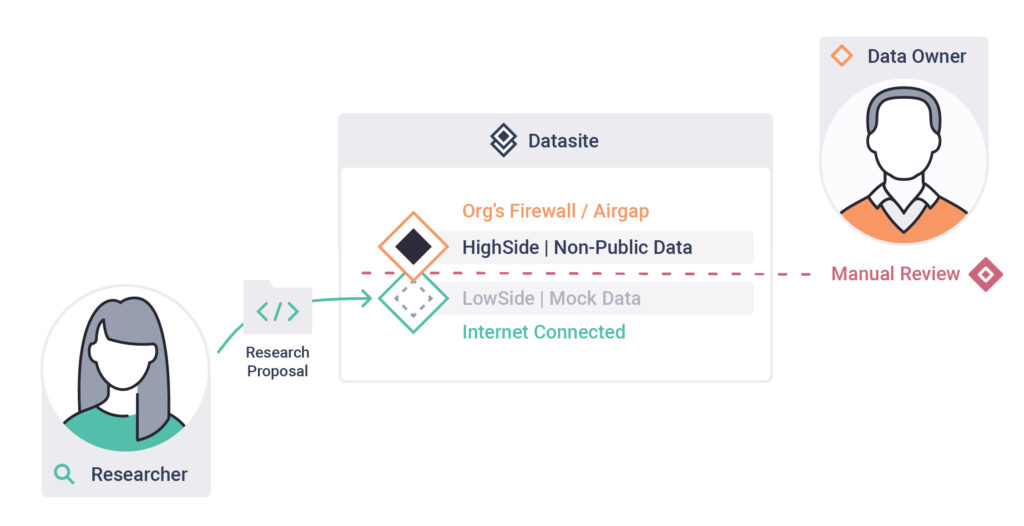
Download Results
Once approved by the datasite owner, download the results of your query.
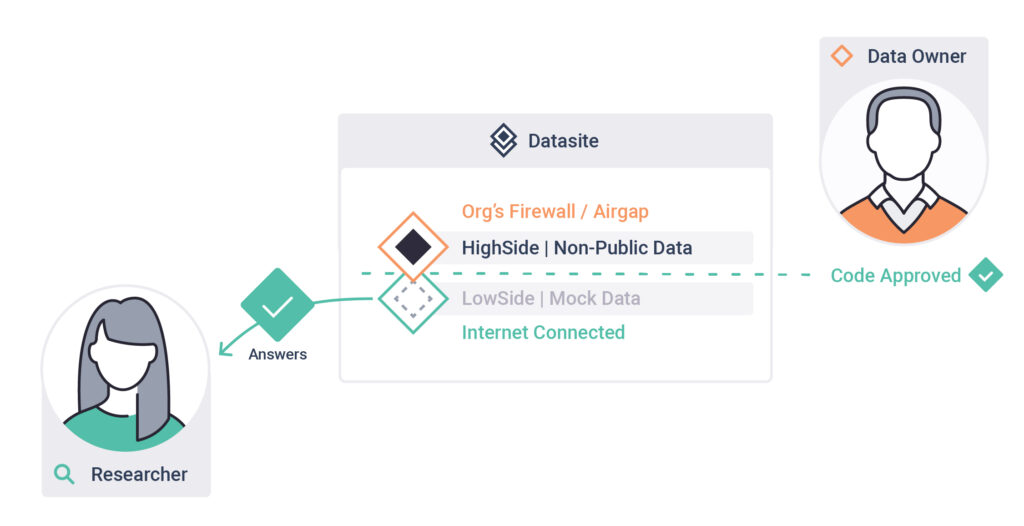
Launch a Secure Datasite
Use Syft’s documentation to set up a datasite and load in your data.
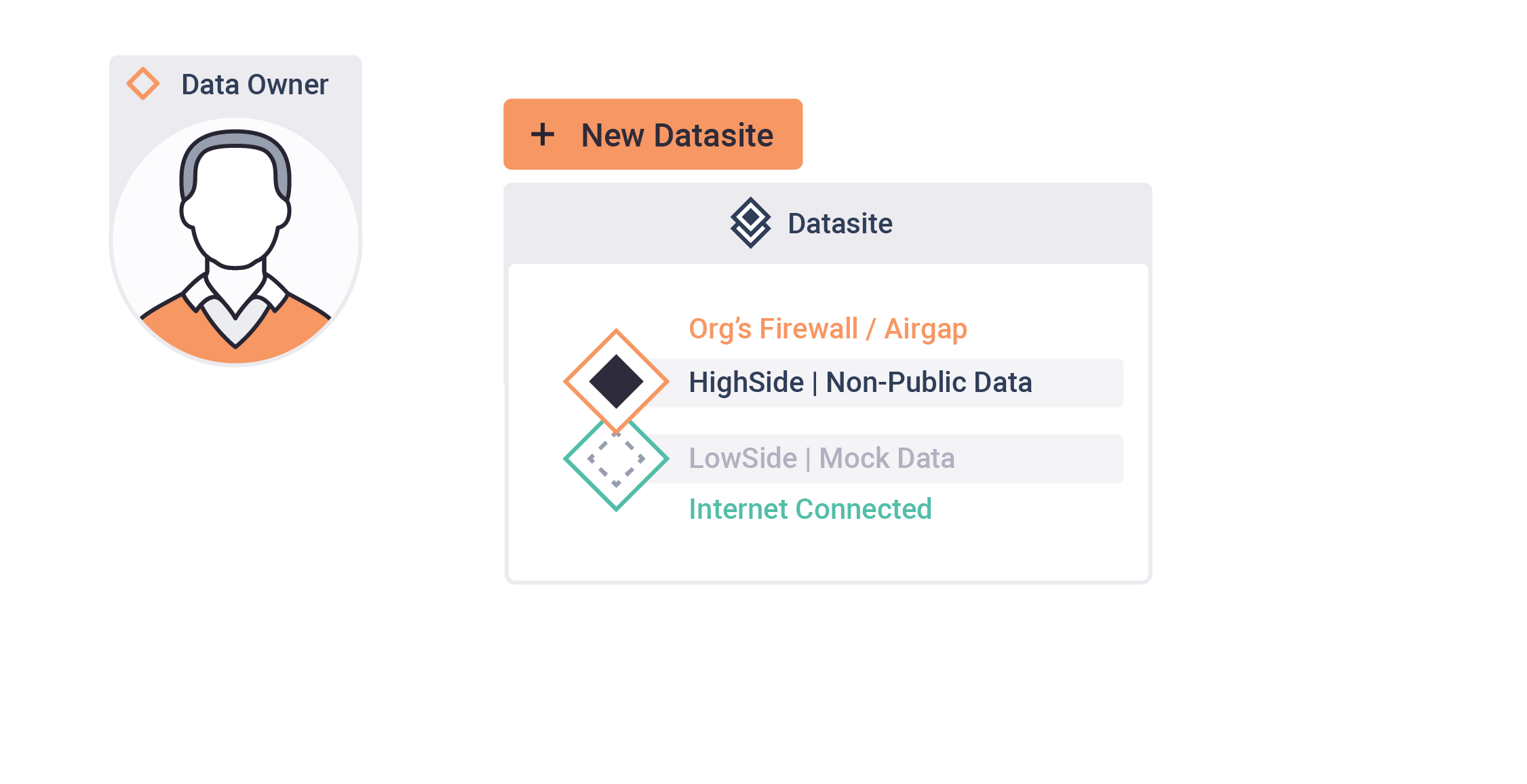
Review Research Proposals
Evaluate research proposals submitted to your datasite ensuring no sensitive data is compromised.
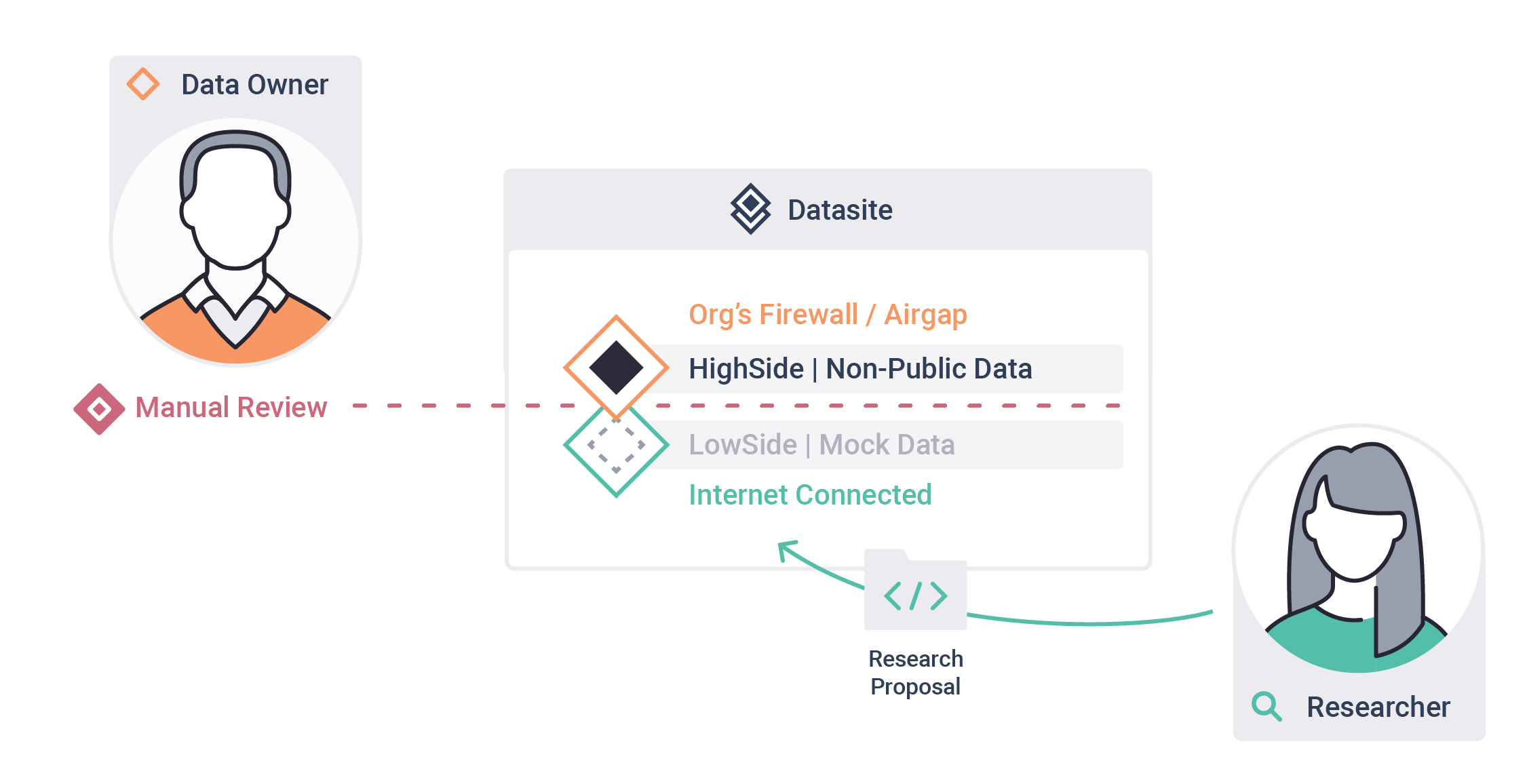
Approve compliant projects
Approve the project once it meets your safety standards, allowing the researcher to access the results.
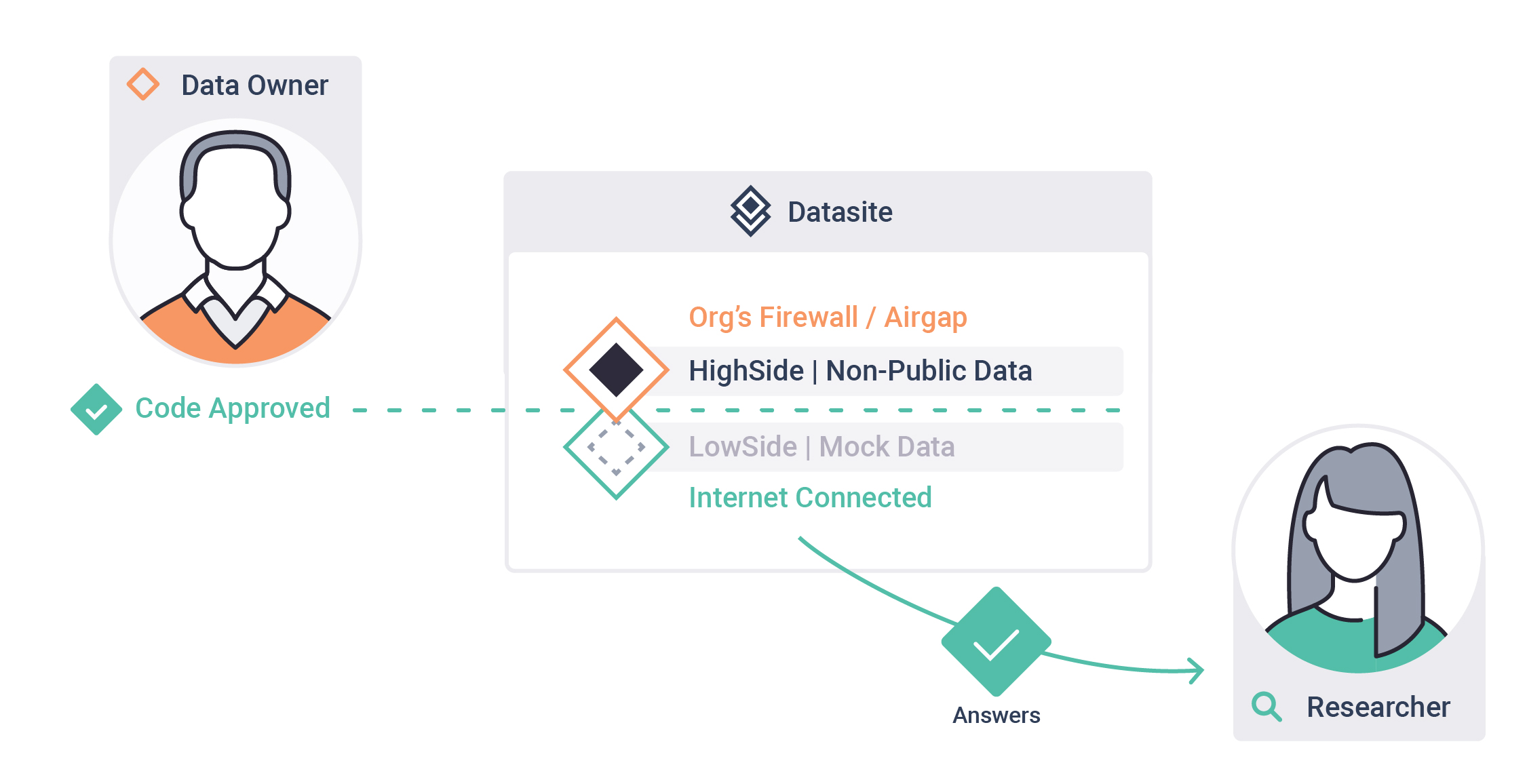
It’s easy to get started
Learn how to get or grant privacy-enhanced access to non-public data.
Challenge your research limits with Syft
Conduct Monumental Research Projects
The Syft Network connects researchers with non-public data at a scale previously unattainable, unlocking massive collaborative project potential.
Get your projects funded by aligned grants
OpenMined can connect objective-aligned researchers and data owners with grants to fund important research projects.
Work with some of the world’s most reputable organizations
The Syft Network enables collaborative remote research with the world’s most powerful and data-rich organizations.
FAQ
How does Syft ensure the analysis doesn’t reveal personal or protected information?
Syft preserves data privacy by enabling data scientists to study data without ever acquiring a copy. In traditional data science flows, researchers see raw private data during the process, which they can copy or remember. Syft avoids this problem, protecting the privacy of the data, through several groundbreaking features it supports:
Mock-Data-Based Prototyping: The first reason researchers have to look at data is that they need something to use to write their research code. Syft gets around this problem by giving researchers that “something” in a way that preserves privacy — a fake/mock version of the real dataset — identical in every way except the actual values of the data are randomized.
Manual Code Review: The second reason researchers have to look at data is because they can run any computation on the data and look at the results. Research/data science is inherently iterative, and researchers really want to be able to iterate. Syft gets around the need for researchers to see the data while preserving their ability to iterate. The simplest way Syft does this is by empowering researchers to submit their code — prototyped against mock data — to the data owner’s staff for review and execution.
Automation Using Privacy-Enhancing Technologies: While the first two features above can (in theory) support any type of researcher accessing any type of dataset without seeing it — it involves researchers waiting for code reviews and data owners spending time reviewing code. Syft overcomes this problem by introducing a variety of privacy-enhancing technologies — access control, federated learning, differential privacy, zero-knowledge proofs, etc. — which enable a data owner to automatically approve some requests, giving researchers instant results and allowing data owners to avoid manual review.
What security measures does Syft implement to ensure that personal or protected information isn’t compromised?
Syft creates solid, traditionally accepted barriers between external researchers and internal computers holding data. In its most secure setting, Syft puts an “air gap” between them, although it can also be configured for a similar “VPN-gapped” configuration.
In these configurations, an internal employee is responsible for moving assets across the “airgap.” Syft provides secure serialization support for objects moving across the “airgap” and convenient triaging to ensure this process is efficient and secure.
In its highest security setting, no research code runs on an organization’s private data without its employees observing every line.
While Syft includes tooling to increase efficiency and provide some automation around the process (approvals, pre-approvals, PETs, etc.), it can do so while upholding this principle.
Where else is Syft deployed?
We have unlocked sensitive user data in places such as Microsoft and GDPR-protected data in areas such as Dailymotion through our partnership with the Christchurch Call Initiative on Algorithmic Outcomes, where external data scientists could perform research on production AI recommender models.
We also have active projects with the US Census Bureau, the Italian National Institute of Statistics (Istat), Statistics Canada (StatCan), and the United Nations Statistics Division (UNSD) to demonstrate how joint analysis across restricted statistical data can work internationally across national statistics offices.
Additionally, we have an active project with the xD team at the US Census Bureau to help make their Title-13 and Title-26 protected data available for research.
We are also the infrastructure of choice for Reddit’s external researcher program.
Press Room
Frontier AI Taskforce: second progress report
The report announced new partnerships with leading AI organizations, bringing UK AISI’s network of partner organizations to eleven. Announced partnerships included UK AISI’s work with OpenMined to develop and deploy technical infrastructure that will facilitate AI safety research across governments and AI research organizations.

From privacy to partnership: The role of PETs in data governance and collaborative analysis
This report, developed in close collaboration with the Alan Turing Institute, considers how PETs could play a significant role in responsible data use by enhancing data protection and collaborative data analysis. It is divided into three chapters covering the emerging marketplace for PETs, the state of standards and assurance, and use cases for PETs. OpenMined’s work and courses are featured in the report.
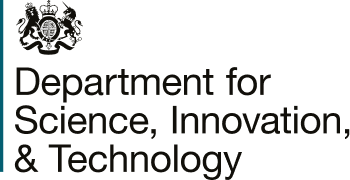
Privacy-preserving third-party audits on Unreleased Digital Assets with PySyft
OpenMined was profiled by the Department for Science, Innovation, and Technology in the United Kingdom in its portfolio of AI assurance techniques for its flagship software, PySyft. PySyft allows model owners to load information concerning production AI algorithms into a server, where an external researcher can send a research question without ever seeing the information in that server.
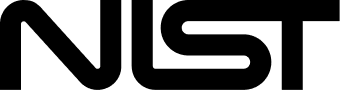
Winners Announced in First Phase of UK-US PETs Prize Challenges
For the first stage of the competition, teams from academic institutions, global technology companies, and privacy tech companies submitted white papers describing their approaches to privacy-preserving data analytics. OpenMined, in partnership with DeepMind, was one of the organizations selected for prizes in the UK.
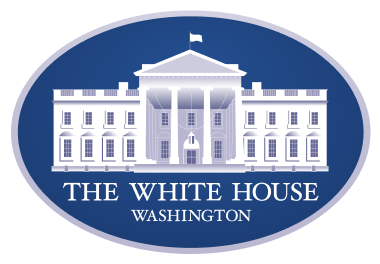
OSTP-NSF-PCAST Event on Opportunities at the AI Research Frontier
The White House Office of Science and Technology Policy (OSTP), the U.S. National Science Foundation (NSF), and the President’s Council of Advisors on Science and Technology (PCAST) convened an event to recognize two artificial intelligence (AI) research and development milestones in the Biden-Harris Administration’s efforts to help leverage the benefits of AI to benefit all of America: the first round of the National AI Research Resource (NAIRR) Pilot awards, and the release of the PCAST report, “Supercharging Research: Harnessing Artificial Intelligence to Meet Global Challenges.” OpenMined was invited to participate in the event.
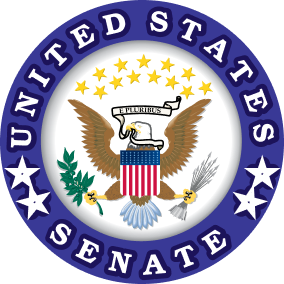
Driving US Innovation in AI: A Roadmap for AI Policy in the US Senate
The Senate formed a Bipartisan AI working Group to bring leading experts into a unique dialogue with the Senate on some of the most profound policy questions AI presents. In doing so, they aimed to help lay the foundation for a better understanding in the Senate of the policy choices and implications around AI. OpenMined was invited to participate in the AI Insight Forum on Transparency, Explainability, Intellectual Property, and Copyright.
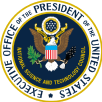
National Strategy to Advance Privacy-Preserving Data Sharing and Analytics
The National Strategy to Advance Privacy-Preserving Data Sharing and Analytics (PPDSA) highlights the US Census Bureau’s pilot project, which deployed OpenMined’s flagship open-source software, PySyft. In this pilot, they tested the ability to query data across a network made up of countries that participate in the United Nations PET Lab without seeing the data itself. The pilot represents one of the first feasibility studies utilizing PPDSA techniques in this way and in an international context, illustrating the effectiveness and capabilities of PPDSA technologies today and encouraging their continued adoption and future potential.
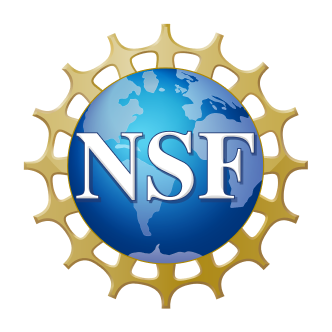
Democratizing the future of AI R&D: NSF to launch National AI Research Resource pilot
OpenMined joined the National Science Foundation (NSF) and partners from across government and industry in launching the National AI Research Resource (NAIRR) pilot — a first step toward realizing a shared vision for a healthy, trustworthy AI ecosystem in the United States. Bringing together computational, data, software, model, training, and user support resources, the NAIRR pilot aims to set the foundation for a shared research infrastructure that will strengthen and democratize access to the critical resources needed to power responsible AI discovery and innovation and maintain the country’s position as a global leader in AI research.
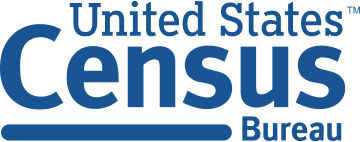
A New Model for International, Privacy-Preserving Data Science
The paper details a proof-of-concept involving using OpenMined’s PySyft to perform a private join on synthetic data representing realistic trade data from UN Comtrade without each NSO needing to access the other NSO’s data directly.
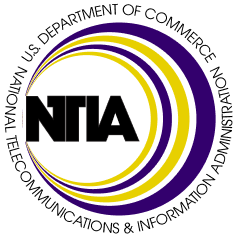
AI Accountability Policy Report
The U.S. Department of Commerce’s National Telecommunications and Information Administration (NTIA) is the President’s principal advisor on telecommunications and information policy issues. In April 2023, the NTIA released a Request for Comment (RFC) on various questions surrounding AI accountability policy. The RFC elicited 1,400+ comments from various stakeholders, including OpenMined. OpenMined’s comments informed the report on its consideration of tradeoffs regarding accountability, appropriate external access to AI systems, using PETs to mitigate privacy risks from third-party access, and standardizing structured transparency as a cost de-escalator.
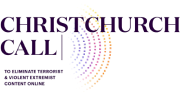
Christchurch Call Initiative on Algorithmic Outcomes
The Christchurch Call Initiative on Algorithmic Outcomes (CCIAO) is a partnership between governments across the world, online platforms, and civil society organizations to invest in accelerated technology development to study algorithmic outcomes independently. OpenMined is the software provider for the CCIAO, developing and testing technical solutions to support the mission of the Call.
Expanding our commitments to countering violent extremism online
Microsoft joined OpenMined, Twitter, and the governments of New Zealand and the United States to research the impact of AI systems that recommend content. The partnership explores how PETs can drive greater accountability and understanding of algorithmic outcomes through proof of function testing of a new kind of research infrastructure.
Investing in privacy enhancing tech to advance transparency in ML
X has partnered with OpenMined to test and explore the potential for PETs at X. X shares OpenMined’s vision of a healthy ecosystem of algorithmic transparency based on an infrastructure of PETs, which could broadly allow for third parties to assess an algorithm’s behavior without exposing either the algorithm or its training data.
Introducing a New Subreddit for Researchers
Reddit desired to preserve public access to Reddit content for researchers and those who believe in responsible non-commercial use of public data. So, they partnered with OpenMined to build out tools and develop a researcher access program to increase the ranks of researchers collaborating with Reddit and each other.
Privacy and Machine Learning Courses
Facebook AI and OpenMined have partnered to offer the Private AI Series, a course that teaches developers about privacy-preserving machine learning (PPML) using PyTorch. This series has two goals: to provide the broadest possible awareness and education around both PPML concepts and hands-on development and to promote key ML and PPML technologies.
Partnering with OpenMined to make differential privacy more widely accessible
Google announced a new partnership with OpenMined to develop a version of its differential privacy library specifically for Python developers. This library will allow developers to access a new and unique way to treat their data with world-class privacy.
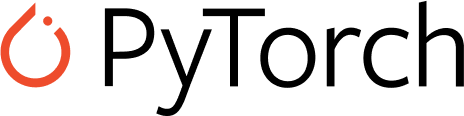
OpenMined and PyTorch partner to launch fellowship funding for privacy-preserving ML community
The PyTorch team has invested $250,000 to support OpenMined in furthering the development and proliferation of privacy-preserving ML.
The PET Guide
The UN Guide on PETs for Official Statistics presents methodologies and approaches to mitigate privacy risks when using sensitive or confidential data. Detailed case studies are presented, including two that feature OpenMined’s work and flagship software.
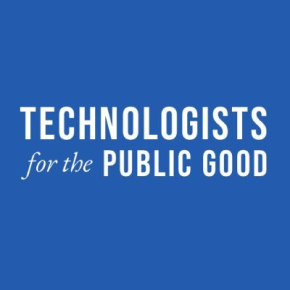
Facilitating Access to Non-Public Data: A World in Which All Data is “Open”
At the Technologists for Public Good Demo Day, Andrew Trask, Executive Director of OpenMined, presented a vision where researchers can safely access non-public data as quickly as using a public website. This is made possible by combining the core strengths of various PETs. PETs offer governments a new tool to bolster their open data policies, enforce their open data regulations and legislations, and continue to increase the quality and quantity of data openly available to the research community.

Reimagining Our High-Tech World
Mike Kubzansky, CEO of Omidyar Network, highlights OpenMined’s work to build out the technical infrastructure to enable full transparency.