Introduction to Attribution-Based Control (ABC)
A New Paradigm for Safe, Trustworthy, Responsible AI
Many of AI’s risks in areas like AI evaluations, privacy, value alignment, copyright, concentration of power, loss of control, and hallucinations can be reduced to the lack of attribution-based control in AI systems, which itself can be reduced to the overuse of addition and copying within AI algorithms. OpenMined’s primary technical goal is to synthesize a handful of recently proposed techniques in deep learning, cryptography, and distributed systems into a viable path to reduce addition and copying, provide attribution-based control, and address many of AI’s primary risks while maximizing its benefits — unlocking 6+ orders of magnitude more data, compute, and associated AI evaluations and capability in the process. This article details the fundamental problem of attribution-based control, and the ways it underpins other problems like privacy, value alignment, copyright, concentration of power, loss of control, hallucinations, and AI evaluations.
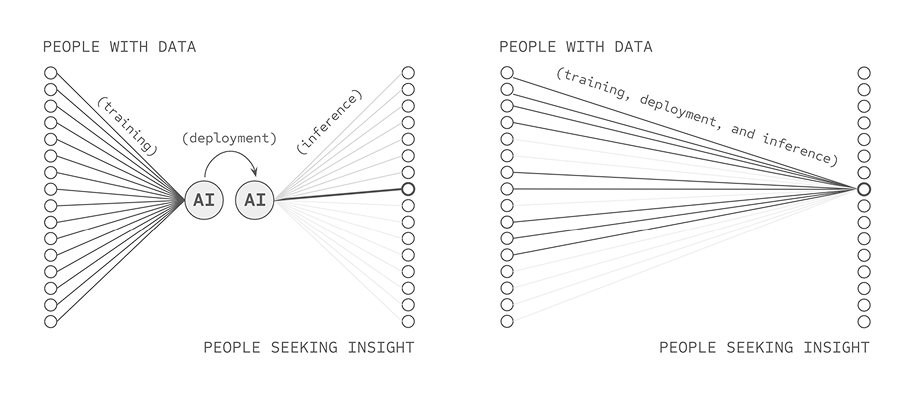
Traditional open/closed-source AI systems (left) centralize data collection and decision-making, while ABC-enabled AI systems (right) enable direct communication between those with data and those seeking insights.
The Problem of Attribution-Based Control (ABC)
Attribution-based control (ABC) describes a relationship between sources of AI-related data, compute, and talent and the AI users receiving predictions. These two groups of people have ABC when two things are true:
- Resource owners: dynamically control how much intelligence to give each AI prediction they support
- AI users: dynamically control which resource providers they want to rely upon for each AI prediction.
While AI systems learn from data, current AI architectures fail to offer resource owners and users attribution-based control—meaning they cannot verify or manage which data sources influence which AI predictions. This has cascading consequences across society at multiple levels: individual, institutional, societal, and geopolitical.
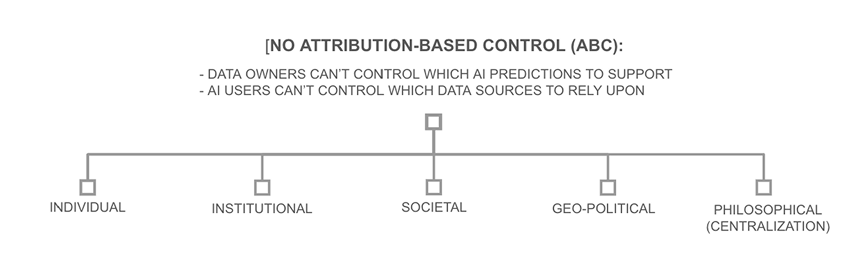
ABC presents problems at several levels of society.
Individual Consequences: Authenticity and Trust
At an individual level, missing ABC leads to authenticity issues—hallucinations, deepfakes, and disinformation. Unlike traditional research methods, AI-generated content typically offers no bibliography or verification mechanism. For instance, a medical student researching rare diseases cannot verify if the AI-sourced information originates from peer-reviewed journals or less reliable sources.
AI “hallucinates” by combining unrelated data points to produce plausible yet incorrect outputs, a problem inherent to the lack of attribution. Similarly, disinformation occurs when AI merges unrelated concepts, like combining celebrity images with controversial acts to produce misleading deepfakes. Without ABC, users cannot identify or correct such misinformation.
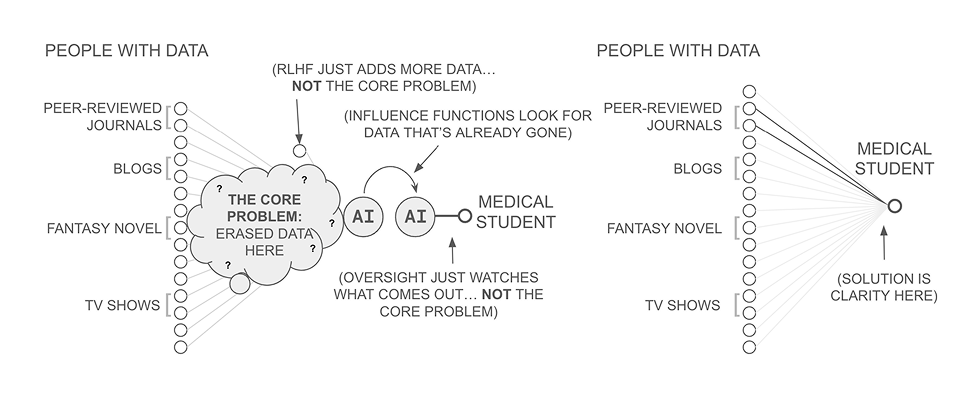
Traditional open/closed-source AI systems (left) mathematically delete information linking source and prediction. Once this information is gone, neither adding more data (RLHF), looking for source information (influence functions), or providing oversight over AI systems addresses the core issue. Instead, ABC-enabled AI systems (right) enable direct communication between those with data and those seeking insights. In ABC-enabled AI, attribution and control flow with the information, enabling verification of sources and protection of contributor interests.
Institutional Consequences: Data Sharing and Control
For institutions holding valuable data, the absence of ABC creates an incentive problem. Institutions must choose between contributing to AI development—risking loss of control and privacy—or withholding data, limiting AI’s capabilities. Major medical institutions, for instance, often decline AI partnerships due to privacy and control concerns, significantly hindering advancements in fields like cancer detection.
Privacy-enhancing technologies (PETs), such as federated learning and secure multi-party computation, address privacy but still fail to deliver precise control over data usage. Without attribution, institutions cannot selectively support or withdraw from specific AI predictions, perpetuating data siloing.
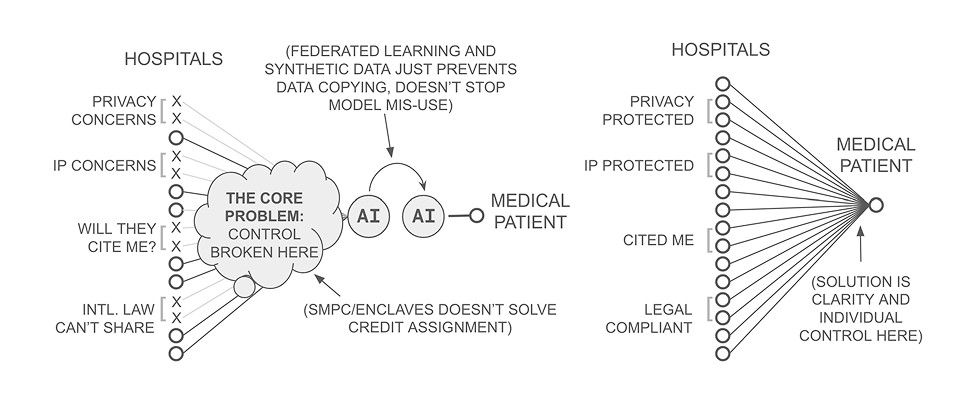
Traditional open/closed-source AI systems (left) copy data to AI providers, giving them unilateral control over the resulting model and its predictions. Instead, ABC-enabled AI systems (right) enable direct communication between those with data and those seeking insights. In ABC-enabled AI, attribution and control flow with the information, enabling data sources to retain control over which predictions they seek to support.
Societal Consequences: Governance and Representation
At a societal level, the absence of ABC creates governance crises—AI safety concerns, value misalignment, and bias. AI systems concentrate control in the hands of their creators or maintainers, leading to decisions misaligned with societal values. Without ABC, AI decisions about content filtering and model behavior are made by a small group, not reflecting the broader public’s interests.
ABC addresses this issue by reconnecting data contributors and AI users, ensuring continuous public consent and representation in AI-driven decisions.
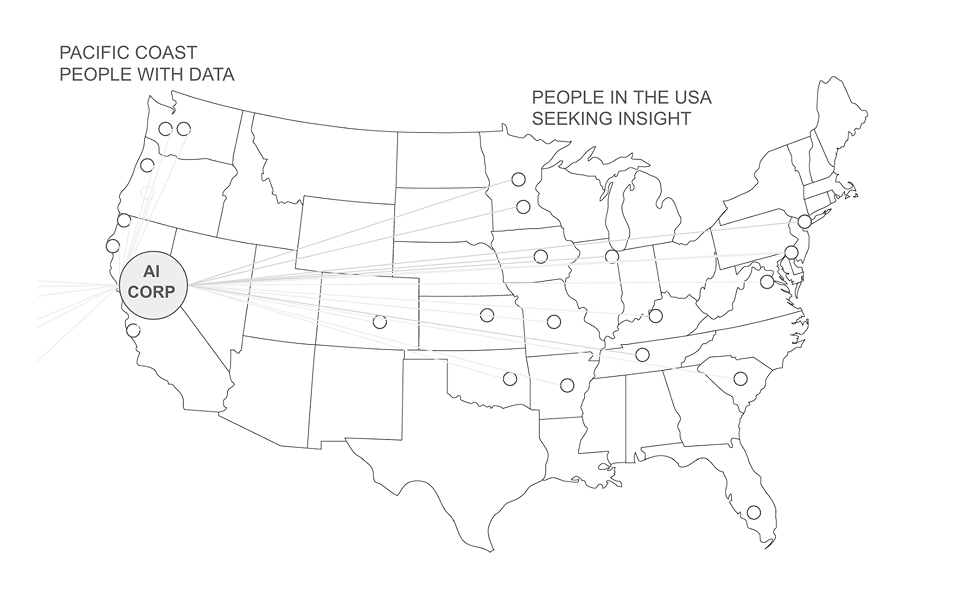
A fictional illustration of an AI model trained on data from people across the US Pacific coast, and used by people in the eastern half of the United States. However, irrespective of where the training data comes from or the predictions go to — a handful of people at the AI organization retains centralized control over the AI model’s capability and use. This calls into question how/whether the AI or its creators will forego selfish incentives and use the AI’s intelligence to serve society broadly.
Geopolitical Consequences: Democracy vs. Centralization
On the geopolitical stage, the lack of ABC creates a stark choice: centralize data and decision-making, compromising democratic values, or lose AI capabilities to authoritarian regimes that do centralize aggressively. This dilemma underpins current geopolitical AI competitions, notably between democratic nations and authoritarian states like China.
Proposals like an “AI Manhattan Project” to centralize resources in democratic nations risk undermining core democratic principles without guaranteeing competitive advantage. ABC provides a decentralized alternative, allowing democratic nations to leverage vast global data and compute resources without centralization.
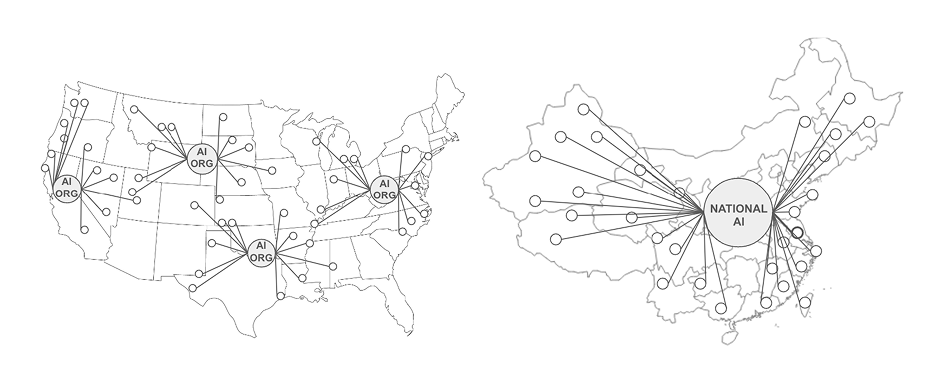
A fictional illustration of a traditional AI model trained within organizations across the US (left) and trained across all organizations in China (right). It is meant to illustrate the difference between resources acquired at the corporation level and at the nation level.
Centralization Consequences: AI as Central Intelligence or Communication Tool
Ultimately, the lack of ABC positions AI as a tool of central control rather than a decentralized communication medium. Current AI systems centralize knowledge, guiding its use according to centralized interests, which disadvantages societies that resist centralization.
By shifting from operations that break ABC (addition and copying) to those that preserve it, AI can evolve into a true communication technology, directly connecting knowledge holders with seekers. This change is not merely technical; it aligns AI development with democratic principles and broad societal interests.
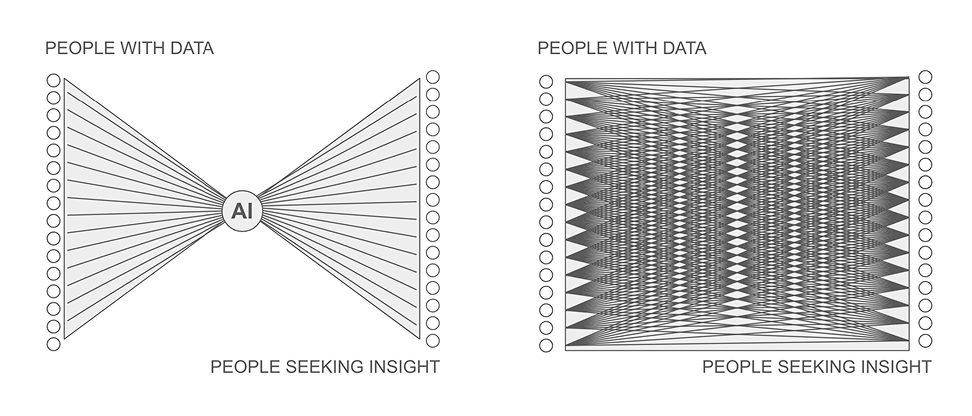
(left) An illustration of AI as an intermediary between those who have information and those who seek insights. (right) An illustration of AI as a communication device.
The Road Ahead: From Deep Learning to Broad Listening
Recent breakthroughs in deep learning, cryptography, and distributed systems have begun addressing these fundamental issues, paving the way toward practical ABC solutions. At OpenMined, we’re seeking to transform those solutions into free, open-source software across a live network: the public network for non-public information.
Attribution-Based Control Updates
Sign up to receive emails that explain this concept in bite-sized ideas, use cases and stories.
Launching Q3, 2025.
This subscribes you to messages From Andrew,
OpenMined’s Executive Director. Opt out any time.